Although time intensive, closing the loop between your customer relationship management (CRM) software and website provides invaluable opportunities to drive your marketing strategy.
With the rising cost of living, consumers are being more discerning with their disposable income. In a more competitive world, products must filter through to consumers, who are placing more expectations on personalisation and getting used to being targeted online.
We were engaged to help one of our automotive clients gain a competitive edge through a sales propensity model. The model required website analytics and the ability to harness this capability between quantification and prediction. The questions they wanted to be answered were:
Sales Propensity Questions:
01.
What does a typical user journey look like after tracing back sales to web history?
02.
What are the best features of a website that buyers gravitate towards? Can we use if for UX?
03.
How to leverage look-a-like audiences?
What is a propensity model?
Before we dive in, lets recap propensity modelling. Propensity modelling uses data and statistical methods to estimate the likelihood of our audience performing specific actions. In the case of marketing, our audience will be consumers or existing customers. The data is usually CRM data or website and app data.
Propensity modelling allows us to create models to predict things like:
Propensity to purchase or convert
Propensity to churn
Predicted customer lifetime value
Propensity to engage
A propensity score allows marketers to group customers for more sophisticated or personalised targeting.
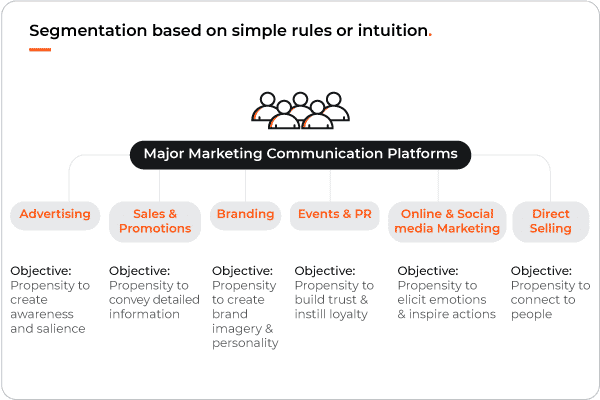
Before propensity modelling we may have the same marketing communications sent to all prospects or customers or they may be segmented by the marketing team based on simple rules or intuition.
.png?)
Through propensity modelling, the prospects or customers are given targeted or personalised marketing communications based on the likelihood to perform desired actions.
How is propensity modelling different to segmentation?
Propensity modelling can be used to create sophisticated segmentation. Basic segmentation groups customers based on historic behaviours or actions. e.g. - a Recency, Frequency, Monetary Value (RFM) segmentation model, whereas propensity modelling focusses on predicting future behaviours or actions to create advanced segments.
How does a bespoke propensity model differ to propensity modelling out of my marketing platform?
Many marketing automation, MarTech, AdTech, personalisation, or customer relationship management (CRM) systems provide propensity modelling Out of the Box (OOTB). A bespoke propensity model allows us to ingest a much greater amount of data to enable more sophisticated predictive modelling. For example, we can connect offline transaction data to enrich CRM data where customers have not scanned their loyalty cards. This is called tokenisation.
Now lets dive in to our approach to propensity modelling for an Australian Automotive client.
Stage 1 - Acknowledging the cliff dive.
Obtaining a valid data sample is increasingly hard to achieve in the present day, so before we commenced work, we established whether the client’s CRM system could be integrated with on-site tagging.
Through analysis of our client’s website traffic (and within industry norms) we found that 80% to 95% of all unique user ids only persisted for 1 day and roughly 10% of IDs persisted for a week or later. The question was, why?
➤ Anonymity: The internet is increasingly becoming more anonymous with regulation.
➤ Mobility: User behaviour has changed through consumers using multiple digital assets and mobile devices to engage with website content. This means that a user may have multiple IDs depending on location and asset change.
While there may be a distinct pool of people researching for their next purchase, our current environment has ensured that these users may have multiple IDs with no way to aggregate them up to single users.
Does this mean that our small pool of successfully traced back buyers is invalid? No, it doesn't. This small pool may represent only less than 5% of all users, but the actual sample itself is highly valid due to the accumulated history and interactions we can study. From there, we can build a model to find the most important behavioural patterns that drive prediction rates and assign look-alikes with probability scores.
Stage 2 – Building the model and recreating the user stories.
Keeping in mind that our clients really wanted to see which pages were being used heavily by buyers from a UX perspective, we built this model by considering what a discerning buyer really wants to research. We then created a hypothesis list about what a car buyer/researcher would want to look at. We settled on a list of 50 hypotheses that include scenarios like:
- “If I were a buyer, I’d like to see this in various colours” or
- “Is a bigger battery better value for money?”
From there, we used each of these hypothesis/scenarios as features in our model and picked an architecture that was simple to interpret for our client.
.png?)
.png?)
To understand how to frame the user journey on the website, it's best to view this chart (Figure 1) which serves to keep things within expectations by illustrating the limitations and constraints of analytics in today’s world.
We used data from past buyers to ascertain which website features were most likely to drive conversions. From there, different model probabilities were generated for a multi layered strategy.
Stage 3 – Leveraging on model results.
Once the model was created, we found the best on-site features to help frame the user journey on the website i.e. - how users found our client’s website and what pages influenced their purchase.
Using Google's in-market targeting, we then categorised users into 4 distinct classes based on probability scores and formulated strategies on what to do with these users in regard to online media.
01. High probability / propensity to purchase
High probability visitors have come to the website often and are actively researching. For such users, personalisation is the best strategy in terms of UX and redirecting them towards the end of the sales funnel on the website. One common example is the “pick up where you left off” prompt when you return to the website, or a “suggestions” page. This is the most cost-effective way to drive conversions by leveraging your website’s assets and UX flow.
Other strategies could flow on from this category, including tailor made ads for these users.
02. Medium probability / propensity to purchase
These are the users who have displayed behaviours that mimic past buyers but have not accumulated enough time or interactions to warrant a “high probability” score. In this case, we leveraged retargeting but with ads that channel them to more “research” based landing pages or re-direction into offer pages.
03. Low probability / on-site / in-market
For low propensity/probability visitors, we tested if they were potentially “in-market” for purchasing on Google’s ecosystem, i.e. - they are very early in their product search but are actively using other competitor websites. In this case, display retargeting would be the best strategy to see if your products can pique their interest as a reconsideration.
04. Low probability
In this case, it was better to serve these users the standard ads and website content as they were very early in their user journey or were casual viewers.
Conclusion
To gain a competitive edge, closing the loop is important between your CRM and website. Despite the small pool of buyers with a stitched back history, propensity modelling will provide invaluable data to inform marketing automation, what is important on-site, improve UX, create the foundations for user personalisation and create an arsenal of options to help bolster your digital marketing strategy.
Resolution Digital has a variety of services to help you get started with GA4:
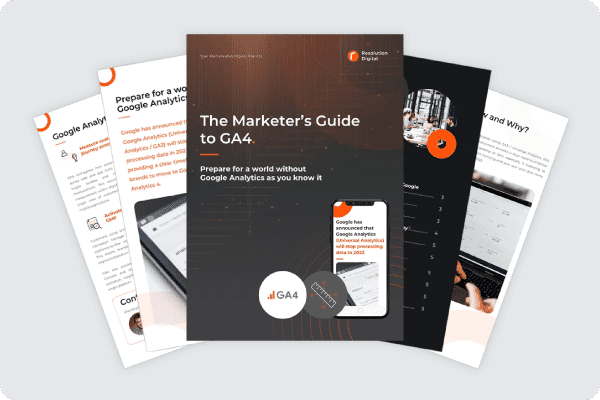
Marketer's Guide to GA4
We created the Marketers Guide to GA4 to help marketers get buy-in from leadership and sign off on the most suitable business case, now. Especially, to collect GA4 data to leverage the new predictive and ML capabilities.
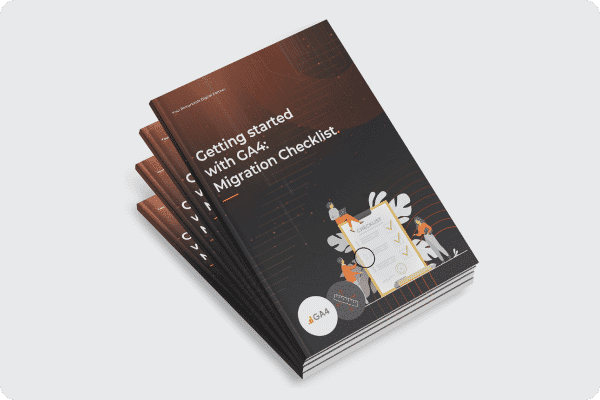
Implementation Checklist
We’ve developed an implementation checklist for in-house teams to manage the deployment of GA4.
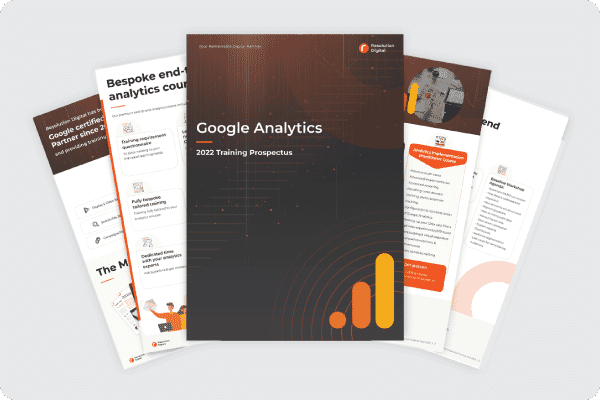
GA4 Training Prospectus
We have just wrapped up our October Advanced Analytics Insights Training course for both public and in-house teams.
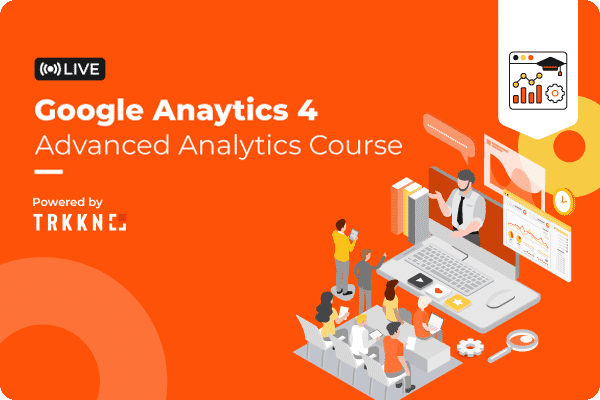
GA4 Advanced Analytics Course
Register your interest to our Google Analytics 4 Advanced Analytics live training course, where you'll learn how to set up properties in GA4, when to use them, and how to use the reports.